Tech that: Size up a crowd the neural way
Convolutional Neural Networks (CNNs) are a subset of neural networks that are better suited to process and recognise images.
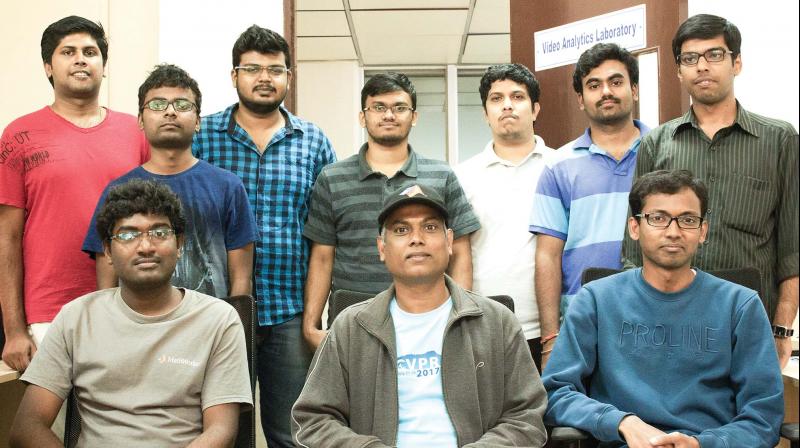
Just how do you count crowds, stand by with an emergency evacuation plan to prevent a catastrophe triggered by a stampede, and all in a few seconds? Technology can help, say experts.
More than 2,400 people have died in crowd disasters in India between 2001 and 2014. In the first Kumbh Mela, post-Independence, in Allahabad in 1954, more than 800 people died and 2,000-odd were injured in a stampede caused when some people pushed through the barricades set up for a procession.
In the subsequent Kumbh Melas and other religious gatherings across the country, a sudden surge by devotees has claimed scores of lives because of lack of crowd control mechanisms and competent management by civic authorities and law enforcement agencies.
Given the fact that so many lives are at stake, how should the civic authorities plan for safety? How do the authorities concerned arrive at a conclusion that there are more people than the security and civic agencies can handle? What would happen in case of a repeat of the blaze at Puttingal Devi temple complex near Kollam, in Kerala, which claimed more than 100 lives last year?
Technology can help manage the crowd and plan for disaster contingency, say scientists at the Indian Institute of Science (IISc), Bengaluru. Prof. Venkatesh Babu and his team at the Department of Computation and Data Sciences have developed a novel method that can accurately estimate the number of people in densely crowded gatherings with the help of neural networks.
Neural networks are computational models inspired by biology that enable a computer to learn from observational data, just like the human brain. They look at thousands of sample data, and learn to recognise patterns. So they are used in applications involving image recognition, speech recognition and natural language processing.
Convolutional Neural Networks (CNNs) are a subset of neural networks that are better suited to process and recognise images. The researchers have tested their method with standard crowd-scene datasets, each containing multiple images of dense and sparse crowd scenes.
“Our method works well for scenes with extremely dense crowds and when the crowd density varies within the scene. This variation in crowd density within a scene is typical of crowded scenes that one might observe in the real world,” says Prof. Venkatesh Babu.
While there are many methods proposed to estimate crowd numbers, the advantage of this method over earlier ones is that it addresses scenes with varying crowd density very well.
“Our technique uses an expert CNN to choose the best CNN from a group of CNNs. This helps it employ different CNNs for different parts of the scene. And our training method achieves this automatically,” explained Prof. Venkatesh Babu.
He said a hand-held device would suffice to gather the data about the crowd, beam it to a high-end Graphics Processing Unit (GPU) and a Central Processing Unit (CPU), and immediately receive an estimate of the size of the gathering.
“Once the size of the crowd is known, security officials can take precautionary measures to avoid a crowd disaster. A GPU costs '60,000, while the other equipment is reasonably priced. So the entire system will not be very expensive,” he added.
Prof. Venkatesh Babu and his team presented their work at the ‘Computer Vision and Pattern Recognition’ (CVPR) conference at Honolulu, Hawaii, US, in July 2017. The team, however, plans to offer the software to a company that can develop a commercial product because “we are an academic research lab and focus on innovation and pushing the limits of performance of our techniques”.
Besides aiding security measures, it could be employed for civic planning, vehicle/traffic management and organisation of events. And, of course, to shoot down controversies like the size of the crowd at the Presidential inauguration of Donald Trump!