Fully automated image recognition software in the works at MIT
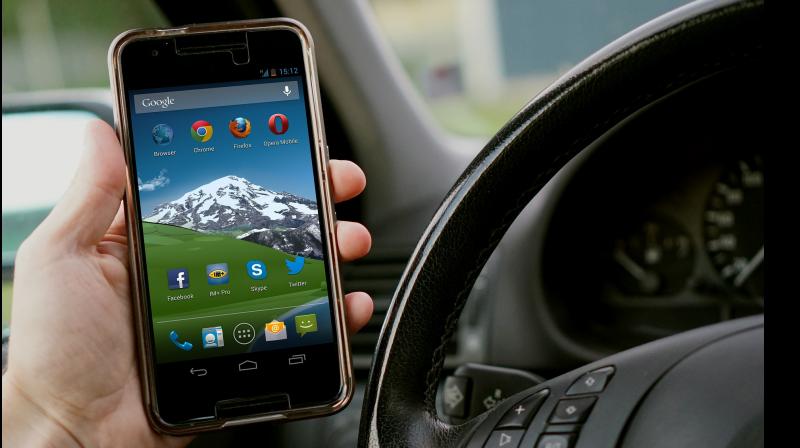
The technology that converts speech to text on cell phones is a lot more complicated that it seems to be. The processor has to scan through a millions of audio files and their transcriptions to identify which acoustic feature matches the stored words. It’s a time-consuming complex procedure, which involves high costs for execution.
Researchers from MIT’s Computer Science and Artificial Intelligence Laboratory (CSAIL) presented a new technology for sound recognition systems at the Information Processing Systems conference this week. This new approach doesn’t need training speech recognition systems to depend on transcriptions; however, it involves a new approach, through which, a system analyses the relation between images and a spoken description of the particular image.
Jim Glass, a senior research scientist at CSAIL and a co-author on the paper describing the new system says, “The goal of this work is to try to get the machine to learn language more like the way humans do.”
“Big advances have been made — Siri, Google — but it’s expensive to get those annotations, and people have thus focused on, really, the major languages of the world. There are 7,000 languages, and I think less than two per cent have ASR [automatic speech recognition] capabilities, and probably nothing is going to be done to address the others. So if you’re trying to think about how technology can be beneficial for society at large, it’s interesting to think about what we need to do to change the current situation. And the approach we’ve been taking through the years is looking at what we can learn with less supervision.”
David Harwath, a graduate in electrical engineering and computer science (EECS) at MIT and Antonio Torralba, an EECS professor joins Jim Glass on the paper.
The idea that has been put forward by the team inculcates a technology where the speech correlates with a group of thematically related images instead of written text. For example, if a speech is associated with a particular group of images, which has text associated with them, then it’s quite possible to find a likely transcription of the speech without human involvement. Since the system senses the word’s meaning and correlates the images associated with them, similar clusters of images will be inferred to have related meanings, like ‘cloud’ and ‘storm’.
The researchers deployed a database of 10,000 images, individually having a recording of a free-form verbal description of it. They would install their system with one of their recording and ask to retrieve 10 best matched images. This set of 10 images would contain the correct one 31 per cent of the time. Their systems were trained from a huge database built by Torralba; Aude Oliva, a principal research scientist at CSAIL and their students. They hired people through Amazon’s Mechanical Turk crowd sourcing site to describe the images verbally with whatever description they could come up with for about 10 to 20 seconds. This kind of testing was necessary to alter the data for best result, though the ultimate aim is to train the system using digital video. “I think this will extrapolate naturally to video,” Glass says.
This system is developed using neural network, which are composed of processing nodes that work like individual neurons, capable of simple computations but connected in dense network. When this neural network is being trained it modifies the operations executed by its nodes to improve its performance. It has two separate networks — one to take images as input and the other that takes spectrograms, representing audio signals as changes of amplitude. The output of the top layer of each network is a 1,024-dimensional vector — a sequence of 1,024 numbers. The final node in the network multiplies the corresponding terms in the vector together and adds them up and produces a single number.
The trained system identifies the dot-product peaks for very spectrogram. The peak picks up the most reliable word that could lead to a perfect image, like a ‘baseball’ in a photo of baseball pitcher in action. Lin-shan Lee, a professor of electrical engineering and computer science at National Taiwan University says, “Possibly, a baby learns to speak from its perception of the environment, a large part of which may be visual. Today, machines have started to mimic such a learning process. This work is one of the earliest efforts in this direction, and I was really impressed when I first learned of it.”
“Perhaps even more exciting is just the question of how much we can learn with deep neural networks,” adds Karen Livescu, an assistant professor at the Toyota Technological Institute at the University of Chicago. “The more the research community does with them, the more we realise that they can learn a lot from big piles of data. But it’s hard to label big piles of data, so it's really exciting that in this work, Harwath et al. are able to learn from unlabeled data. I am really curious to see how far they can take that.”