IIIT Hyd researchers make ML give voice to speech impaired
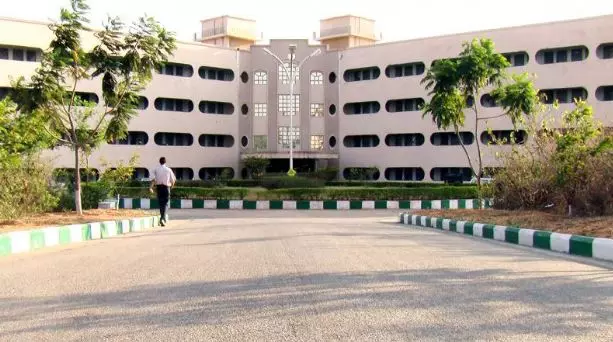
Hyderabad: IIIT Hyderabad researchers have used machine learning (ML) models to help people with speech impairments to generate intelligible speech output. The researchers used the minimalist design of a wireless stethoscope that converts behind-the-ear vibrations –- heard as non-audible whispers — into intelligible speech.
The findings were included in a research paper titled ‘StethoSpeech: Speech generation through a clinical stethoscope attached to the skin’, prepared by a team led by Neil Shah, a TCS researcher and PhD student at the Centre for Visual Information Technology (CVIT), IIITH. The other researchers were Neha Sahipjohn and Vishal Tambrahalli and the team was supervised by Dr Ramanathan Subramanian and Prof. Vineet Gandhi.
They experimented with a silent speech interface (SSI) that can convert non-audible speech into a vocalised output. SSI is a form of communication where an audible sound is not produced. “The most popular and simplest of SSI techniques is lip reading,” Neil said.
Some of the other SSI techniques include ultrasound tongue imaging, real-time MRI (rtMRI), electromagnetic articulography and electropalatography, where vibrations across the vocal folds are analysed to comprehend articulation. According to the researchers, these techniques fall short due to their extreme invasive nature (like coils attached to the lips and tongue for measuring movement) and don’t work in real-time.
The team used a stethoscope attached to the skin behind the ear to convert behind-the-ear vibrations into intelligible speech. “Such vibrations are referred to as non-audible murmurs (NAM)”, said Prof. Gandhi.
The IIITH team curated a dataset of NAM vibrations collected under noisy conditions. These vibrations were paired along with their corresponding text. “We asked people to read out some text – all while murmuring. We captured the vibrations behind their ears while they read out the text. We used that data and trained our model to then convert these vibrations into speech,” said Prof. Gandhi.
The NAM vibrations were transmitted via the stethoscope onto a mobile phone through bluetooth and clear speech was obtained as output on the phone speaker. “We demonstrated that NAM vibrations into speech can happen even in a ‘zero-shot’ setting, which means that it works even for novel speakers whose data has not been used for training the model,” explained Neil. Translating a 10-second NAM vibration takes less than 0.3 seconds and even works well with movement such as when the user is walking.
Users can also choose ethnicity, (like English spoken with a South Indian accent) and gender of the voice. With just four hours of murmuring data recorded of any person, a specialised model can be built just for that person.
The quality of the output is very high. “Most ML algorithms directly convert text into speech but that’s not how humans learn to speak. Newborns first interact with audio, and directly start speaking,” said Prof. Gandhi. To mimic natural speech, the team first built a speech-to-speech system. Then they mapped the sound representation to text, instead of directly going from text to speech like other ML models.
The system has great future implications, with a major advantage being that one can make any speaker ‘speak’ in any language. It can also be used in high-noise environments like a rock concert where even normal speech is unintelligible. It can also be used to decipher discreet communication such as by security guards.