ML model switcher helps monitor real-time traffic
Real-time traffic monitoring systems rely on ML models to process video feeds and detect objects like vehicles and pedestrians.
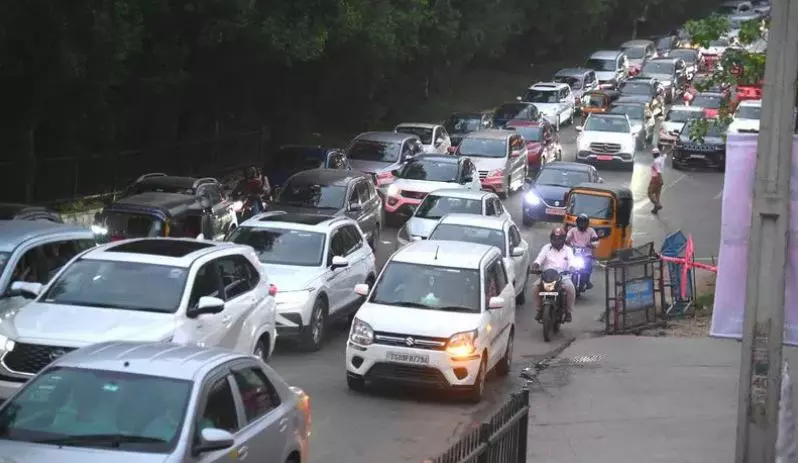
Hyderabad: A group of second-year computer science students has created a machine learning (ML) approach that dynamically switches models on smartphones for real-time traffic monitoring. Their research, developed at the International Institute of Information Technology, Hyderabad (IIIT-H), has been accepted for presentation at an international software architecture workshop in Denmark.
Real-time traffic monitoring systems rely on ML models to process video feeds and detect objects like vehicles and pedestrians. However, choosing the right model is crucial for balancing accuracy, speed, and energy efficiency, particularly on resource-limited devices like smartphones. That’s where Kriti Gupta, Ananya Halgatti, Priyanshi Gupta, and Larissa Lavanya’s innovation comes in. Their method, called EdgeML Balancer, enables edge devices to intelligently switch between different ML models based on real-world conditions.
The idea took shape in the Embedded Systems Workshop, a hands-on course at IIIT-H that encourages learning by doing. “We wanted to work on something different, and we had heard a lot about Qualcomm’s new developer kits,” said Halgatti. These qualcomm innovators development kits built on Snapdragon processors became the foundation for their project.
The team, under the mentorship of Prof. Karthik Vaidyanathan and PhD researcher Akhila Matathammal, said it focused on model switching — a method that selects the most suitable ML model depending on factors like accuracy, response time, and energy consumption. The approach was tested both in simulated scenarios and on real traffic data using a Samsung Galaxy M21 smartphone. “In cloud computing, we optimise for speed and accuracy. But on edge devices, we must also consider power consumption,” explained Matathammal.
What began as an experiment turned into a research paper, “EdgeML Balancer: A Self-Adaptive Approach for Dynamic Model Switching on Resource-Constrained Devices”, which will be presented at the SARECS 2025 workshop in Denmark. The work is now being further refined for testing on the Samsung S24 Ultra
and will be showcased at IIIT-H’s R&D event in March.
Prof. Vaidyanathan said he sees this as proof that undergraduate coursework can lead to significant real-world impact. “This is beyond just grades. It’s international recognition. I hope it inspires other students to look beyond CGPA scores,” he said.